How To
This section contains examples of usual operations in RxSci and Maki Nage. You can find the associated code for most of them here.
Import and Export Data
Read a CSV file
You can work directly on CSV files (versus using Kafka as a source and sink), via the load_from_csv factory operator.
First, import the csv module that contains all CSV related operators:
import rxsci.container.csv as csv
Then you must declare the schema of the CSV file. Below is the schema of the iris dataset that you can retrieve from kaggle.
iris_parser = csv.create_line_parser(
dtype=[
('id', 'int'),
('sepal_length_cm', 'float'),
('sepal_width_cm', 'float'),
('petal_length_cm', 'float'),
('petal_width_cm', 'float'),
('species', 'str'),
]
)
The create_line_parser operator supports options to customize the parser, such as the text encoding, column separator, and default values. See the documentation for more information.
iris_data = csv.load_from_file('./Iris.csv', iris_parser)
Expose a connector
The previous section explained how to read a CSV file directly. This is typically done in a notebook. It is trivial to expose this source of data as a Maki Nage connector, and use it from the makinage CLI.
A source connector is implemented as a function that returns an observable. So the reading of a CSV file can be implemented this way:
def read_iris_from_csv(filename):
return csv.load_from_file(filename, iris_parser)
This function is then declared as a source in the Maki Nage configuration file:
sources:
iris_csv_source:
factory: my_app.connector:read_iris_from_csv
kwargs:
filename: "./Iris.csv"
Write a csv file
The dump_to_file operator writes each input item to a row of a CSV file. The input items must be namedtuples. First, ensure that the data is structured as a namedtuple:
IrisFeature = namedtuple('IrisFeature', ['id', 'species', 'sepal_ratio', 'petal_ratio'])
iris_features = iris_data.pipe(
rs.ops.map(lambda i: IrisFeature(
id=i.id, species=i.species,
sepal_ratio=i.sepal_length_cm / i.sepal_width_cm,
petal_ratio=i.petal_length_cm / i.petal_width_cm
)),
)
The dump_to_file operator uses the fields of the namedtuple to infer the columns names of the CSV file:
iris_features.pipe(
csv.dump_to_file('iris_features.csv', encoding='utf-8'),
).subscribe()
Expose a connector
To use this code in a Maki Nage application, you would expose it as a sink connector. This is done in a similar way than source connectors, except that the connector function returns an operator instead of an observable. Here is the implementation to write a CSV file:
def write_to_csv_file(filename):
return rx.pipe(
csv.dump_to_file(filename, encoding='utf-8'),
)
This function is then declared as a sink in the Maki Nage configuration file:
sinks:
csv_file_sink:
factory: my_app.connector:write_to_csv_file
kwargs:
filename: "./iris_features.csv"
Create Operators
Create an operator by composition
The simplest way to create a new operator is by composing other existing operators. Let’s consider these three operations done on some text input:
rs.ops.map(lambda i: i.replace("-", " "))
rs.ops.filter(lambda i: 'bill' not in i)
rs.ops.map(lambda i: i.capitalize())
The natural way to use them is by chaining them in a pipe:
data.pipe(
rs.ops.map(lambda i: i.replace("-", " "))
rs.ops.filter(lambda i: 'bill' not in i)
rs.ops.map(lambda i: i.capitalize())
)
But you add as more and more data transforms you can end up with a very long pipe. You can quickly improve the readability and reuse some operations by grouping operators. For example, by grouping the previous three operators into a custom operator:
def cleanup_text():
return rx.pipe(
rs.ops.map(lambda i: i.replace("-", " ")),
rs.ops.filter(lambda i: 'bill' not in i),
rs.ops.map(lambda i: i.capitalize()),
)
The function cleanup_text is an operator that you can use in a pipe:
data = [
'hello',
'the-quick-brown-fox',
'bill is fast',
'lorem ipsum'
]
rx.from_(data).pipe(
cleanup_text()
).subscribe(on_next=print)
Hello
The quick brown fox
Lorem ipsum
Create a stateful operator by composition
Stateful operators are more complex to implement because they need to update a state. Hopefully, In many cases, you can create new operators by combining three base operators: scan, filter, and map:
The scan operator updates the state.
The filter operator controls when to emit items.
The map operator emits the items from the state.
Let’s consider the following need: Sum all items up to some threshold. An item must be emitted each time the sum would cross the threshold. Then the sum process starts again:
The state logic can be implemented with the following function:
def _sum_split(acc, i):
if acc[0] + i > threshold:
return (i, acc[0])
return (acc[0]+i, None)
Here acc contains the state. It is a tuple where:
The first field is the current sum
The second field is the item to emit or None if nothing must be emitted.
The full implementation of the operator simply consists in combining scan, filter, and map in a wrapper function:
def sum_split(threshold):
def _sum_split(acc, i):
if acc[0] + i > threshold:
return (i, acc[0])
return (acc[0]+i, None)
return rx.pipe(
rs.ops.scan(_sum_split, seed=(0, None)),
rs.ops.filter(lambda i: i[1] is not None),
rs.ops.map(lambda i: i[1]),
)
You can now use sum_split just as any builtin operator:
data = [1, 2, 3, 4, 1, 2, 6, 1]
rx.from_(data).pipe(
sum_and_split(5)
).subscribe(print)
3 3 5 2 6
Use the configuration parameters
The operators of a Maki Nage application have access to the configuration settings. The configuration is passed as the first argument of the operator. It is an Observable that emits configuration objects. These objects are dicts and they correspond to the content of the YAML configuration file. If the configuration file is read locally, then only one item is emitted. However if the configuration file is served from consul, then one item is emitted each time a change is made in consul.
So the application can choose to use the initial configuration for its whole life, or to dynamically adapt to changes in the configuration.
Use only the initial configuration
Using only the initial configuration is the easiest way to use the configuration settings. This way of working means that the application must be restarted to take into account changes in the configuration file.
The following code shows such an implementation:
def my_operator(config, data):
initial_config = config.pipe(
ops.take(1),
)
result = initial_config.pipe(
ops.flat_map(lambda c: data.pipe(
# my operations, whith c variable available in this scope
rs.ops.map(lambda i: i + c['config']['increment_value'])
)
)
return result,
In this example, the initial_config observable is first created. This observable emits only the first configuration item. Then the computation graph is started from this configuration item, and wrapped in a flat_map scope. Within the scope of flat_map, all operators have access to the c variable. This variable is the dict corresponding to the configuration file.
See this marble diagram for a visual explanation each step:
Use the configuration dynamically
Since the configuration argument is an Observable, it is possible to dynamically adjust the application behavior without having to restart it.
This mode of operation requires that the source items are combined with the configuration items. Then operations can be done on these tuples of item/configuration. Here is the same example that on the previous section, but with the lastest configuration being always used to do the computation:
def my_operator(config, data):
result = data.pipe(
rs.ops.with_latest_from(config),
rs.ops.starmap(lambda i, c: i + c['config']['increment_value']),
)
return result,
See this marble diagram for a visual explanation each step:
Create Connectors
Maki Nage exposes a simple way to write IO connectors. Such connectors allow to read streams of data from any source and to write them to any sink. This is the easiest way to use Maki Nage on data that is not on Kafka. It is also a way to use Maki Nage completely outside of Kafka.
There are two kinds of connectors:
sources
sinks
Source connectors read data from an external source and make it available to the Maki Nage operators.
Sink connectors write data to an external source. They allow exporting the results of your operators.
Here is how connectors are integrated in Maki Nage:
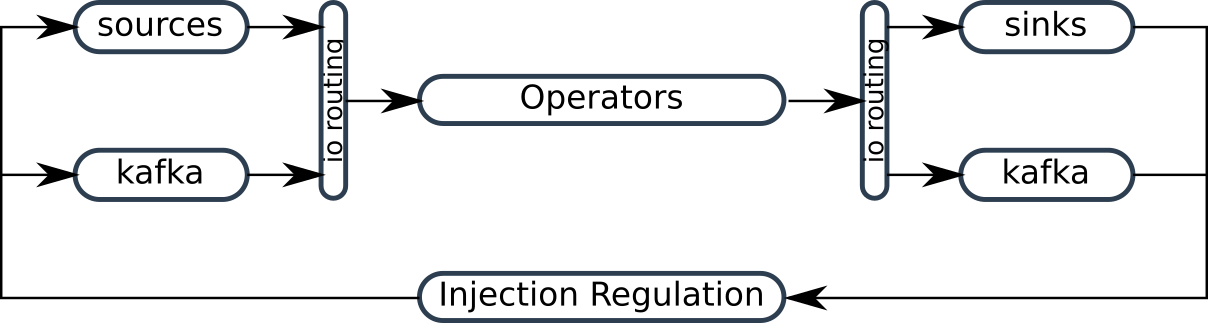
The integration of connectors in Maki Nage guarantees a good integration in Maki Nage. This especially important for the AsyncIO event loop and the backpressure handling.
Moreover connectors can be implemented in dedicated python packages. This encourages the community to share are reuse existing connectors.
Source Connectors
A source connector is implemented as a function that returns an observable. Here is the source used in the house consumption example:
def read_house_data(filename):
data = file.read(filename, size=64*1024).pipe(
line.unframe(),
ops.skip(1),
)
return data
The function read_house_data is the implementation of a source connector. It accepts a parameter: filename. This parameter is provided in the configuration file. This example reads a text file and emits one item per line, excluding the first one.
Sources are subscribed on a dedicated worker thread. As a consequence, they can rely on blocking IO without impacting the rest of the application. Moreover, regulation injection is automatically done on sources when it is enabled.
One declares a connector in the configuration file. The sources section contains the declarations of source connectors. Here is the configuration file used to inject the CSV dataset in Kafka on the house consumption example:
application:
name: push_house_data
kafka:
endpoint: "localhost"
topics:
- name: house_values
encoder: makinage.encoding.string
sources:
house_data:
factory: makinage_examples.house.push:read_house_data
kwargs:
filename: "/opt/dataset/HomeC.csv"
regulators:
- feedback: house_values
control: house_data
operators:
forward_house_data:
factory: makinage_examples.house.push:forward_house_data
sources:
- house_data
sinks:
- house_values
The house_data source is declared in the sources section. Its data come from the read_house_data connector. The arguments of the connectors are set in the kwargs field. This source is then used in the operators the same way as all sources.
Sink Connectors
A sink connector is implemented as a function that returns a ReactiveX operator. Here is the sink used in the house consumption example:
def print_to_console():
return rx.pipe(
ops.do_action(
on_next=print,
on_error=lambda e: print(e),
on_completed=lambda: print("completed")
),
)
In this example, the sink takes no parameters. However, you can add some if needed, the same way as for the source connector. The result of the function is a ReactiveX operator. In this case, it is the result of the rx.pipe function.
Items forwarded to sinks are scheduled on a dedicated worker thread. As a consequence, the sinks can rely on blocking IO without impacting the rest of the application. Moreover, the monitoring of sinks is done automatically when the regulation injection is enabled.
One declares a connector in the configuration file. The sinks section contains the declaration of sink connectors. Here is the configuration file used to print the features computed on the house consumption example:
application:
name: pull_house_data
kafka:
endpoint: "localhost"
topics:
- name: house_features
encoder: makinage.encoding.json
start_from: beginning
sinks:
console:
factory: makinage_examples.house.pull:print_to_console
regulators:
- feedback: console
control: house_features
operators:
forward_house_features:
factory: makinage_examples.house.pull:forward_house_features
sources:
- house_features
sinks:
- console
The console sink is declared in the sinks section. It uses the print_to_console connector. There is no argument in this connector. Should there be some, they would be set in the kwargs field. This sink is then used in the operators the same way as all sinks.